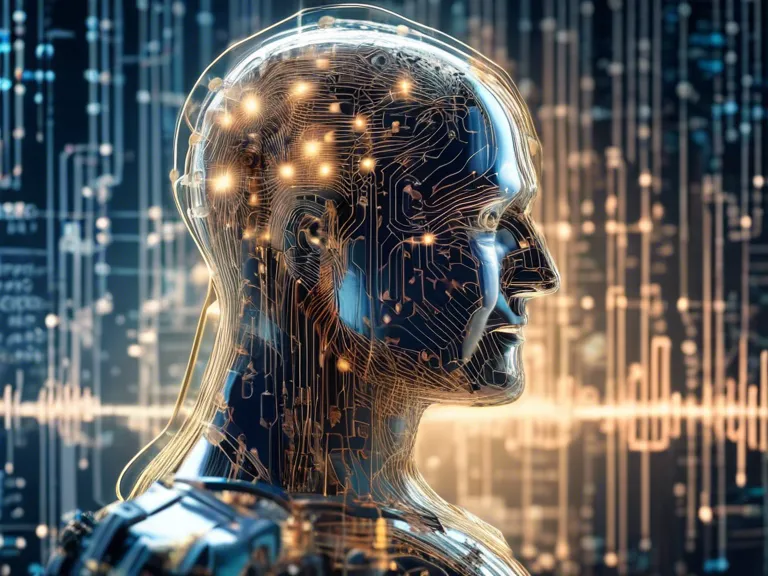
Machine learning solutions are revolutionizing the way cryptocurrency analysis is performed. By leveraging advanced algorithms and data analysis techniques, machine learning models can help investors and traders make more informed decisions in the highly volatile and complex cryptocurrency market. In this article, we will explore how machine learning is being applied to cryptocurrency analysis and discuss the benefits and challenges of using these technologies.
Understanding Cryptocurrency Analysis
Cryptocurrency analysis involves studying various factors that can affect the price and performance of digital assets. Traditional methods of analysis include technical analysis, fundamental analysis, and sentiment analysis. However, with the rapid growth of the cryptocurrency market and the increasing complexity of digital assets, traditional methods may not always provide accurate insights.
Benefits of Machine Learning in Cryptocurrency Analysis
Machine learning offers several advantages for cryptocurrency analysis:
Predictive Modeling: Machine learning models can analyze historical price data and identify patterns and trends that can help predict future price movements.
Risk Management: By analyzing market data and identifying potential risks, machine learning models can help investors develop more effective risk management strategies.
Sentiment Analysis: Machine learning models can analyze social media, news articles, and other sources of information to gauge market sentiment and identify potential market trends.
Automated Trading: Machine learning algorithms can be used to develop automated trading strategies that can execute trades based on predefined criteria without human intervention.
Machine Learning Techniques for Cryptocurrency Analysis
Several machine learning techniques can be applied to cryptocurrency analysis, including:
Regression Analysis: Regression models can help predict the future price of cryptocurrencies based on historical data.
Classification: Classification models can help identify patterns in market data and classify assets based on certain criteria.
Clustering: Clustering algorithms can group cryptocurrencies based on similarities in price movements, market capitalization, or other factors.
Deep Learning: Deep learning techniques, such as neural networks, can analyze large volumes of data and identify complex patterns that may not be apparent through traditional analysis methods.
Challenges of Using Machine Learning in Cryptocurrency Analysis
While machine learning offers many benefits for cryptocurrency analysis, there are also challenges to consider:
Data Quality: Cryptocurrency market data can be noisy and unreliable, which can affect the performance of machine learning models.
Overfitting: Overfitting occurs when a model performs well on historical data but fails to generalize to new data. This can be a significant challenge in the cryptocurrency market, where trends can change rapidly.
Interpretability: Machine learning models can be complex and difficult to interpret, making it challenging for investors to understand the reasoning behind certain predictions.
Conclusion
Machine learning solutions are transforming the way cryptocurrency analysis is conducted, offering valuable insights and predictive capabilities to investors and traders. By leveraging advanced algorithms and data analysis techniques, machine learning models can help navigate the complexities of the cryptocurrency market and make more informed decisions. While there are challenges to overcome, the benefits of using machine learning in cryptocurrency analysis are undeniable, paving the way for a more data-driven and efficient approach to investing in digital assets.