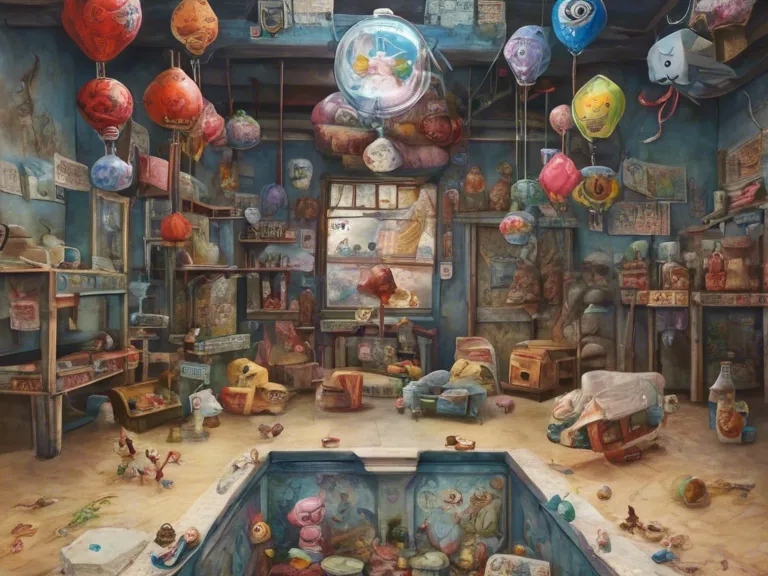
Understanding the Importance of 'Where' in Machine Learning
Machine learning is a field of artificial intelligence that focuses on developing algorithms and models that allow computers to learn from and make predictions or decisions based on data. One crucial aspect of machine learning is understanding the concept of 'where,' which plays a significant role in various applications and algorithms.
Spatial Data and Geospatial Analysis
In machine learning, the concept of 'where' often refers to spatial data, which includes information related to geographical locations, coordinates, and maps. Geospatial analysis is a key area where the 'where' plays a vital role. By utilizing geospatial data, machine learning algorithms can identify patterns, trends, and relationships that are specific to certain locations.
Location-Based Recommendations and Personalization
Location-based recommendations and personalization are common applications of 'where' in machine learning. By analyzing a user's location data, preferences, and behavior, algorithms can provide personalized recommendations for services, products, or content based on their geographic context. This approach enhances user experience and improves the relevance of recommendations.
Spatial Clustering and Classification
Spatial clustering and classification are techniques used in machine learning to group data points based on their spatial proximity or similarities. By considering the 'where' aspect of the data, algorithms can identify clusters or classes that exhibit spatial relationships, leading to more accurate predictions and insights.
Spatial-Temporal Analysis and Predictive Modeling
In many real-world scenarios, understanding both the spatial and temporal aspects of data is crucial for making accurate predictions and decisions. Machine learning models that incorporate spatio-temporal analysis can forecast future trends, events, or behaviors by considering not just 'what' is happening but also 'where' and 'when' it is occurring.
Applications in Transportation, Urban Planning, and Environmental Science
The integration of 'where' in machine learning has revolutionized various industries, including transportation, urban planning, and environmental science. By leveraging spatial data and advanced algorithms, stakeholders can optimize routes, plan infrastructure developments, and monitor environmental changes with precision and efficiency.
Conclusion
The concept of 'where' in machine learning is a fundamental aspect that underpins many applications and algorithms. By incorporating spatial data, geospatial analysis, and location-based insights, machine learning models can deliver more accurate predictions, personalized recommendations, and valuable insights across various domains. Understanding the importance of 'where' is essential for harnessing the full potential of machine learning in solving complex real-world problems.
applebyandwood.com
auzigog.com
eac-w.com
homesbyelevation.com
nihilismforoptimists.com
slavonkandhortus.com
thekoreanpolitics.com
turningpointpt.com
val-up.com
wakansen.com
3dideation.com
achilles-fire.com
banatelhalal.com
biyografirehberi.com
bohams.com
comisiondeestudios.com
cooride-net.com
danayumul.com
ecadecom.com
edwardscornerfarmersmarket.com
ekspresif.com
ellajmae.com
ginroecooks.com
gracefueled.com
hightidekitchen.com
jeroenswolfs.com
marthalott.com
mollybroekman.com
mpthoidai.com
plumfashionpr.com
racktents.com
solzapower.com
southcoastbehavioralhealth.com
the101bali.com
thearguide.com
theartistfia.com
thefitnesswire.com
thelivelihoodproject.com
thelynndentonagency.com
wilkespoolsnspas.com
wjwatson.com
drinkganbei.com
mendenhallnews.com
nathaliemoliavko-visotzky.com
nationalinfertilityday.com
wide-aware.com
ashleymodernfurniture.com
babylonbusinessfinance.com
charliedewhirst.com
christianandmilitaryhats.com
hypnosisoneonone.com
icelandcomedyfilmfestival.com
kayelam.com
mlroadhouse.com
mumpreneursonline.com
posciesa.com
pursweets-and.com
rgparchive.com
therenegadehealthshow.com
travelingbitz.com
yutakaokada.com
22fps.com
aarondgraham.com
essentialaustin.com
femdotdot.com
harborcheese.com
innovar-env.com
mercicongo.com
oabphoto.com
pmptestprep.com
rmreflectivevest-jp.com
tempistico.com
filmintelligence.org
artisticbrit.com
avataracademyagency.com
blackteaworld.com
healthprosinrecovery.com
iancswanson.com
multiversecorpscomics.com
warrenindiana.com
growthremote.com
horizonbarcelona.com
iosdevcampcolorado.com
knoticalpr.com
kotaden.com
la-scuderia.com
nidoderatones.com
noexcuses5k.com
nolongerhome.com
oxfordcounselingcenter.com
phytacol.com
pizzaropizza.com
spotlightbd.com
tenbags.com
thetravellingwilbennetts.com
archwayintl.com
jyorganictea.com
newdadsplaybook.com
noahlemas.com
qatohost.com
redredphoto.com
rooms4nhs.com
seadragonenergy.com
spagzblox.com
toboer.com
aumantvmuseum.com
beyondausten.com
citylabstudio.com
diskonio.com
drinkcf.com
eft-dongle.com
emilymeganphotography.com
evolveathleticclub.com
godleystationvet.com
hirochanweb.com
homeonefurniture.com
ifiwasastylist.com
lacantinepopup.com
liriklagubatak.com
lo-ko.com
mensagenseatividades.com
myway-zeus.com
nevadadec.com
nokarikhabar.com
nuuuki.com
quenchpad.com
sckyrock.com
tindunghanoi.com
tradeshows-biz.com
wikimuzik.com