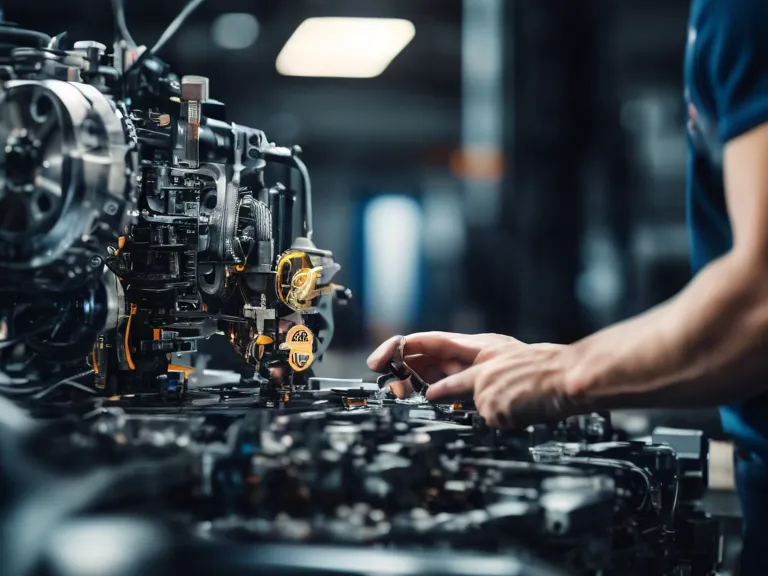
Machine learning is revolutionizing many industries, including mechanics, by enabling predictive maintenance solutions that can help prevent costly equipment breakdowns. However, managing and monitoring these machine learning applications can be complex and time-consuming. One way to streamline this process is by automating maintenance tasks within machine learning applications in mechanics.
### Importance of Maintenance in Mechanics
Maintenance is a critical aspect of the mechanics industry as it ensures that equipment and machinery are functioning efficiently and reliably. Traditional maintenance practices often rely on scheduled inspections and reactive repairs, which can be costly and inefficient. By incorporating machine learning algorithms into maintenance practices, organizations can shift towards a predictive maintenance approach, where equipment failures can be anticipated and prevented before they occur.
### Challenges in Managing Machine Learning Applications
While machine learning algorithms can provide valuable insights into equipment performance and predict potential failures, managing and monitoring these applications can be challenging. The continuous monitoring of data streams, updating of models, and deployment of predictive maintenance strategies require significant time and expertise. This can strain resources and limit the scalability of machine learning applications in mechanics.
### Benefits of Automating Maintenance Tasks
Automating maintenance tasks within machine learning applications can offer several benefits. By automating data collection, preprocessing, model training, and deployment processes, organizations can streamline their maintenance workflows and improve operational efficiency. Automation can also help reduce human errors, ensure consistency in maintenance practices, and free up resources to focus on more strategic tasks.
### Implementation of Automation in Maintenance
To automate maintenance tasks within machine learning applications, organizations can leverage tools and technologies such as workflow automation platforms, data pipelines, and cloud computing services. These tools can help automate repetitive tasks, schedule maintenance workflows, and integrate machine learning models seamlessly into existing systems. By implementing automation in maintenance, organizations can enhance the accuracy and reliability of predictive maintenance solutions.
### Best Practices for Automating Maintenance
When automating maintenance tasks within machine learning applications, organizations should follow some best practices to ensure successful implementation. This includes defining clear objectives for automation, establishing robust data pipelines, monitoring model performance regularly, and integrating feedback loops for continuous improvement. Collaborating with domain experts and data scientists can also help optimize maintenance automation solutions for specific industry requirements.
### Conclusion
Automating maintenance tasks within machine learning applications in mechanics can enhance the efficiency, accuracy, and scalability of predictive maintenance solutions. By leveraging automation tools and best practices, organizations can streamline their maintenance workflows, reduce operational costs, and improve equipment reliability. Embracing automation in maintenance is essential for staying competitive in the evolving mechanics industry and maximizing the benefits of machine learning applications.
Source:
askchrisknight.com
askchristopherknight.com
berkshirefoodjournal.com
cchirosw.com
coastalcottagesnl.com
destinationsite.com
finulldraft.com
gardenstatepaintingnj.com
impacthound.com
inneddi.com
itazurakoneko.com
judyforaz.com
localcarsnow.com
lovedayscinema.com
matiqistiqomahsambas.com
meetborofive.com
morskicovik.com
naturesrewardphotography.com
regenerativeresorts.com
restaurant-nashvilletn.com
scoopsicecreamtruck.com
simmonsmotorworks.com
trinityeco-tours.com
uncoverstudios.com
wong-multimedia.com
123exp-government.com
abozymes.com
atsheatingandair.com
bemjavim.com
caseintrend.com
cdnxbvn.com
charangolibre.com
denis-latin.com
frugal-science.com
fukuoka-kouyaren.com
hanabisou.com
herculessportcenter.com
highvoltageloja.com
hlwstoryboard.com
juderabig.com
leauxandbehold.com
melurix-next.com
moggiechicken.com
mytrendysins.com
nativeskinonline.com
ninethreestudio.com
pcmwereld.com
pichinkufibers.com
primerautobody.com
salmanhatta.com
shalevbrosh.com
sosorryrecords.com
sportsone-egy.com
wofome.com
ycadeau.com
abalawpc.com
airportpawnmiami.com
alchemycollaborative.com
blackfigdesigns.com
cubemedica.com
deliciousyarns.com
denledamtrancaocap.com
denledpanelcaocap.com
futebolrico.com
honsyuji.com
jayasupranaschool.com
jstact.com
kikichaos.com
knuckleupmartialarts.com
lavi-furniture.com
maisonsecomatiq.com
neighbors-construction.com
nspointers.com
opticiwear.com
richouli.com
soddydaisyhealthcare.com
soultalkmagazine.com
studywithpmouy.com
swordfightsandstarrynights.com
tamatur-bali.com
thepainreliefsecret.com
townhouselinens.com
vchainstore.com
vormaro.com
yukonlodgeforsale.com
airkoolny.com
an-geeke.com
bajka3.com
bertinoargenti.com
birolkitabevi.com
burikoland.com
emeraldseamless.com
floridanativecharters.com
iptvsportt.com
jupiterboutique.com
kateronline.com
kustomkoachrv.com
lademeuredufondateur.com
lucianamurta.com
luckydelaware.com
luckyindiana.com
lujannavas.com
mike4rep.com
milioshairsalon.com
movingrestaurantequipment.com
openseedvault.com
retrogradetours.com
revistaresiduos.com
roughandreadygrange.com
therusalka.com
toxnfill3.com
valleymaintenancelandscape.com
violetorlandi.com
wanpaku-golf.com
zinkdental.com
suryacctv.com
tuvannugioi.com
mandalynmusic.com
wireless-reviews.com
tussingblockwatch.com
greenteaphotography.com
jennierooney.com
vietamreview.net
kyavar.com
market-truth.com
alexandregaurier.com
omegagadget.com
banditosla.com
nursememama.com
oyunbilim.com
acme-nuclear.com
agilityimap.com
akikcombong.com
anniesmysteries.com
bflofoodie.com
brandzonestudios.com
cacemar.com
daperezlaw.com
denvyautomation.com
eugeneband.com
factory-eshop.com
florentdumas.com
hishaywireless.com
in2-signs.com
kuwekeza-holdings.com
mitaniya-ltd.com
mixfoure.com
mobilitypluspro2.com
moipravila.com
montreal-business-kit.com
mortiseandmiter.com
nextdigitaldental.com
nurdalilahputri.com
oem-phoneaccessories.com
palmbeachestepona.com
precavida.com
roscoeandetta.com
scriptsnmacros.com
sringserver.com
thecustomfairy.com
withlovefromangela.com
applebyandwood.com
auzigog.com
eac-w.com
homesbyelevation.com
nihilismforoptimists.com
slavonkandhortus.com
thekoreanpolitics.com
turningpointpt.com
val-up.com
wakansen.com
3dideation.com
achilles-fire.com
banatelhalal.com
biyografirehberi.com
bohams.com
comisiondeestudios.com
cooride-net.com
danayumul.com
ecadecom.com
edwardscornerfarmersmarket.com
ekspresif.com
ellajmae.com
ginroecooks.com
gracefueled.com
hightidekitchen.com
jeroenswolfs.com
marthalott.com
mollybroekman.com
mpthoidai.com
plumfashionpr.com
racktents.com
solzapower.com
southcoastbehavioralhealth.com
the101bali.com
thearguide.com
theartistfia.com
thefitnesswire.com
thelivelihoodproject.com
thelynndentonagency.com
wilkespoolsnspas.com
wjwatson.com
drinkganbei.com
mendenhallnews.com
nathaliemoliavko-visotzky.com
nationalinfertilityday.com
wide-aware.com
ashleymodernfurniture.com
babylonbusinessfinance.com
charliedewhirst.com
christianandmilitaryhats.com
hypnosisoneonone.com
icelandcomedyfilmfestival.com
kayelam.com
mlroadhouse.com
mumpreneursonline.com
posciesa.com
pursweets-and.com
rgparchive.com
therenegadehealthshow.com
travelingbitz.com
yutakaokada.com
22fps.com
aarondgraham.com
essentialaustin.com
femdotdot.com
harborcheese.com
innovar-env.com
mercicongo.com
oabphoto.com
pmptestprep.com
rmreflectivevest-jp.com
tempistico.com
filmintelligence.org
artisticbrit.com
avataracademyagency.com
blackteaworld.com
healthprosinrecovery.com
iancswanson.com
multiversecorpscomics.com
warrenindiana.com
growthremote.com
horizonbarcelona.com
iosdevcampcolorado.com
knoticalpr.com
kotaden.com
la-scuderia.com
nidoderatones.com
noexcuses5k.com
nolongerhome.com
oxfordcounselingcenter.com
phytacol.com
pizzaropizza.com
spotlightbd.com
tenbags.com
thetravellingwilbennetts.com
archwayintl.com
jyorganictea.com
newdadsplaybook.com
noahlemas.com
qatohost.com
redredphoto.com
rooms4nhs.com
seadragonenergy.com
spagzblox.com
toboer.com
aumantvmuseum.com
beyondausten.com
citylabstudio.com
diskonio.com
drinkcf.com
eft-dongle.com
emilymeganphotography.com
evolveathleticclub.com
godleystationvet.com
hirochanweb.com
homeonefurniture.com
ifiwasastylist.com
lacantinepopup.com
liriklagubatak.com
lo-ko.com
mensagenseatividades.com
myway-zeus.com
nevadadec.com
nokarikhabar.com
nuuuki.com
quenchpad.com
sckyrock.com
tindunghanoi.com
tradeshows-biz.com
wikimuzik.com
askchrisknight.com
askchristopherknight.com
berkshirefoodjournal.com
cchirosw.com
coastalcottagesnl.com
destinationsite.com
finulldraft.com
gardenstatepaintingnj.com
impacthound.com
inneddi.com
itazurakoneko.com
judyforaz.com
localcarsnow.com
lovedayscinema.com
matiqistiqomahsambas.com
meetborofive.com
morskicovik.com
naturesrewardphotography.com
regenerativeresorts.com
restaurant-nashvilletn.com
scoopsicecreamtruck.com
simmonsmotorworks.com
trinityeco-tours.com
uncoverstudios.com
wong-multimedia.com
123exp-government.com
abozymes.com
atsheatingandair.com
bemjavim.com
caseintrend.com
cdnxbvn.com
charangolibre.com
denis-latin.com
frugal-science.com
fukuoka-kouyaren.com
hanabisou.com
herculessportcenter.com
highvoltageloja.com
hlwstoryboard.com
juderabig.com
leauxandbehold.com
melurix-next.com
moggiechicken.com
mytrendysins.com
nativeskinonline.com
ninethreestudio.com
pcmwereld.com
pichinkufibers.com
primerautobody.com
salmanhatta.com
shalevbrosh.com
sosorryrecords.com
sportsone-egy.com
wofome.com
ycadeau.com
abalawpc.com
airportpawnmiami.com
alchemycollaborative.com
blackfigdesigns.com
cubemedica.com
deliciousyarns.com
denledamtrancaocap.com
denledpanelcaocap.com
futebolrico.com
honsyuji.com
jayasupranaschool.com
jstact.com
kikichaos.com
knuckleupmartialarts.com
lavi-furniture.com
maisonsecomatiq.com
neighbors-construction.com
nspointers.com
opticiwear.com
richouli.com
soddydaisyhealthcare.com
soultalkmagazine.com
studywithpmouy.com
swordfightsandstarrynights.com
tamatur-bali.com
thepainreliefsecret.com
townhouselinens.com
vchainstore.com
vormaro.com
yukonlodgeforsale.com
airkoolny.com
an-geeke.com
bajka3.com
bertinoargenti.com
birolkitabevi.com
burikoland.com
emeraldseamless.com
floridanativecharters.com
iptvsportt.com
jupiterboutique.com
kateronline.com
kustomkoachrv.com
lademeuredufondateur.com
lucianamurta.com
luckydelaware.com
luckyindiana.com
lujannavas.com
mike4rep.com
milioshairsalon.com
movingrestaurantequipment.com
openseedvault.com
retrogradetours.com
revistaresiduos.com
roughandreadygrange.com
therusalka.com
toxnfill3.com
valleymaintenancelandscape.com
violetorlandi.com
wanpaku-golf.com
zinkdental.com
suryacctv.com
tuvannugioi.com
mandalynmusic.com
wireless-reviews.com
tussingblockwatch.com
greenteaphotography.com
jennierooney.com
vietamreview.net
kyavar.com
market-truth.com
alexandregaurier.com
omegagadget.com
banditosla.com
nursememama.com
oyunbilim.com
acme-nuclear.com
agilityimap.com
akikcombong.com
anniesmysteries.com
bflofoodie.com
brandzonestudios.com
cacemar.com
daperezlaw.com
denvyautomation.com
eugeneband.com
factory-eshop.com
florentdumas.com
hishaywireless.com
in2-signs.com
kuwekeza-holdings.com
mitaniya-ltd.com
mixfoure.com
mobilitypluspro2.com
moipravila.com
montreal-business-kit.com
mortiseandmiter.com
nextdigitaldental.com
nurdalilahputri.com
oem-phoneaccessories.com
palmbeachestepona.com
precavida.com
roscoeandetta.com
scriptsnmacros.com
sringserver.com
thecustomfairy.com
withlovefromangela.com
applebyandwood.com
auzigog.com
eac-w.com
homesbyelevation.com
nihilismforoptimists.com
slavonkandhortus.com
thekoreanpolitics.com
turningpointpt.com
val-up.com
wakansen.com
3dideation.com
achilles-fire.com
banatelhalal.com
biyografirehberi.com
bohams.com
comisiondeestudios.com
cooride-net.com
danayumul.com
ecadecom.com
edwardscornerfarmersmarket.com
ekspresif.com
ellajmae.com
ginroecooks.com
gracefueled.com
hightidekitchen.com
jeroenswolfs.com
marthalott.com
mollybroekman.com
mpthoidai.com
plumfashionpr.com
racktents.com
solzapower.com
southcoastbehavioralhealth.com
the101bali.com
thearguide.com
theartistfia.com
thefitnesswire.com
thelivelihoodproject.com
thelynndentonagency.com
wilkespoolsnspas.com
wjwatson.com
drinkganbei.com
mendenhallnews.com
nathaliemoliavko-visotzky.com
nationalinfertilityday.com
wide-aware.com
ashleymodernfurniture.com
babylonbusinessfinance.com
charliedewhirst.com
christianandmilitaryhats.com
hypnosisoneonone.com
icelandcomedyfilmfestival.com
kayelam.com
mlroadhouse.com
mumpreneursonline.com
posciesa.com
pursweets-and.com
rgparchive.com
therenegadehealthshow.com
travelingbitz.com
yutakaokada.com
22fps.com
aarondgraham.com
essentialaustin.com
femdotdot.com
harborcheese.com
innovar-env.com
mercicongo.com
oabphoto.com
pmptestprep.com
rmreflectivevest-jp.com
tempistico.com
filmintelligence.org
artisticbrit.com
avataracademyagency.com
blackteaworld.com
healthprosinrecovery.com
iancswanson.com
multiversecorpscomics.com
warrenindiana.com
growthremote.com
horizonbarcelona.com
iosdevcampcolorado.com
knoticalpr.com
kotaden.com
la-scuderia.com
nidoderatones.com
noexcuses5k.com
nolongerhome.com
oxfordcounselingcenter.com
phytacol.com
pizzaropizza.com
spotlightbd.com
tenbags.com
thetravellingwilbennetts.com
archwayintl.com
jyorganictea.com
newdadsplaybook.com
noahlemas.com
qatohost.com
redredphoto.com
rooms4nhs.com
seadragonenergy.com
spagzblox.com
toboer.com
aumantvmuseum.com
beyondausten.com
citylabstudio.com
diskonio.com
drinkcf.com
eft-dongle.com
emilymeganphotography.com
evolveathleticclub.com
godleystationvet.com
hirochanweb.com
homeonefurniture.com
ifiwasastylist.com
lacantinepopup.com
liriklagubatak.com
lo-ko.com
mensagenseatividades.com
myway-zeus.com
nevadadec.com
nokarikhabar.com
nuuuki.com
quenchpad.com
sckyrock.com
tindunghanoi.com
tradeshows-biz.com
wikimuzik.com