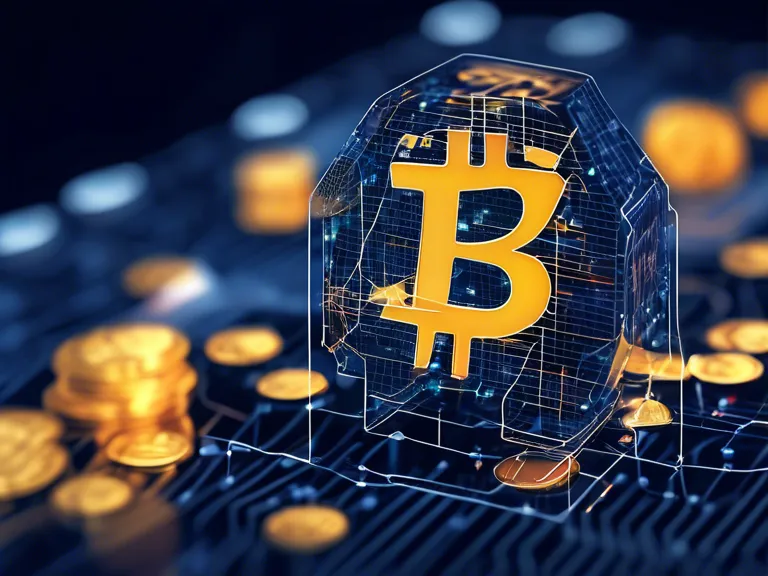
Introduction
Cryptocurrency markets are known for their high volatility, making it challenging for investors to predict price movements. However, with the advancements in machine learning (ML) techniques, predictive modeling has become increasingly popular in the cryptocurrency market. By leveraging historical data and ML algorithms, investors can make more informed decisions and potentially increase their profits. In this article, we will explore the concept of predictive modeling in the cryptocurrency market with ML and how it can be used to forecast price trends.
Understanding Predictive Modeling
Predictive modeling is the process of using historical data to make predictions about future outcomes. In the context of the cryptocurrency market, predictive modeling involves analyzing various factors such as price movements, trading volume, market sentiment, and external events to forecast the direction of prices. ML algorithms play a crucial role in this process by identifying patterns and trends in the data that can be used to make accurate predictions.
Importance of Predictive Modeling in Cryptocurrency Market
The cryptocurrency market operates 24/7 and is highly influenced by factors such as news events, regulatory changes, and market sentiment. Traditional methods of analysis may not always be effective in such a dynamic environment. Predictive modeling with ML offers a data-driven approach that can adapt to changing market conditions and provide more accurate forecasts.
ML Techniques for Predictive Modeling
There are several ML techniques that can be used for predictive modeling in the cryptocurrency market, including:
Linear Regression
Linear regression is a simple yet powerful ML algorithm that can be used to establish relationships between variables and predict future values based on historical data. In the cryptocurrency market, linear regression can be applied to predict price movements based on factors such as trading volume and market capitalization.
Time Series Analysis
Time series analysis is a specialized technique for modeling time-dependent data, making it particularly useful for forecasting price trends in the cryptocurrency market. By analyzing historical price data and identifying patterns, time series analysis can help predict future price movements with a high degree of accuracy.
Sentiment Analysis
Sentiment analysis involves analyzing social media, news articles, and other sources of information to gauge market sentiment and investor behavior. ML algorithms can be used to process large volumes of text data and extract valuable insights that can be used to predict price trends in the cryptocurrency market.
Challenges and Considerations
While predictive modeling with ML can offer valuable insights into the cryptocurrency market, there are several challenges and considerations to keep in mind:
- Data Quality: The accuracy of predictions depends on the quality of the data used for training the ML models. It is essential to ensure that the data is clean, accurate, and representative of market conditions.
- Overfitting: Overfitting occurs when a model performs well on training data but fails to generalize to new, unseen data. Regular validation and testing are necessary to prevent overfitting and ensure the reliability of predictions.
- Market Volatility: Cryptocurrency markets are highly volatile, making them inherently unpredictable. ML models may struggle to capture sudden price movements or unexpected events, leading to inaccurate forecasts.
Conclusion
Predictive modeling in the cryptocurrency market with ML offers a data-driven approach to forecasting price trends and making informed investment decisions. By leveraging historical data and ML algorithms, investors can gain valuable insights into market dynamics and potentially increase their profitability. While there are challenges and considerations to navigate, the use of predictive modeling in the cryptocurrency market is likely to continue growing as technology advances and investors seek more sophisticated tools for analysis.